FinOps for AI: Navigating the Wild West of Generative AI Costs
Buckle up, folks! The rapid evolution of cloud services and the rise of generative AI are reshaping how organizations approach technology adoption in 2024. As businesses saddle up for this wild ride, they’ve got to balance the game-changing potential of AI with the challenges of wrangling costs and complexity in an ever-shifting environment. In this no-holds-barred exposé, CloudBolt explores the key trends shaping cloud service adoption, focusing on how generative AI is shaking things up and what you need to do to stay ahead of the pack.
Generative AI: The New Gold Rush
Generative AI, powered by large language models like GPT-4 and PaLM, is driving a significant shift in cloud adoption patterns. The immense computational requirements of these models, often relying on specialized hardware like GPUs and TPUs, are pushing businesses to leverage cloud infrastructure to access the necessary resources without substantial upfront investments.
Cloud providers have responded by offering AI-as-a-Service (AIaaS) solutions, democratizing access to powerful AI capabilities. Cloud providers, always ready to seize an opportunity, have also rolled out AI-as-a-Service (AIaaS) solutions, making AI accessible to the masses. But hold onto your wallets—the insatiable appetite of AI workloads is driving up cloud spending, forcing companies to get creative and adopt new cost management strategies.
According to McKinsey’s podcast “Rewiring for the era of gen AI,” only about 10% of companies are realizing significant value from generative AI so far in 2023, with many getting stuck in “death by a thousand pilots” without scaling impact. This highlights the importance of effectively managing costs and scaling AI initiatives to drive real business value.
The Good, the Bad, and the Costly: AI Workload Management
According to Forrester’s Technology & Security Predictions 2025, many enterprises will prematurely scale back AI investments due to ROI pressure, with 49% expecting ROI within 1-3 years and 44% within 3-5 years.
The dynamic nature of AI workloads presents unique challenges for cost management in the cloud. The pay-as-you-go model, while offering flexibility, can lead to unpredictable expenses as AI workloads require dynamic scaling based on fluctuating demand. Over-provisioning resources or underestimating capacity needs can result in either wasted spend or performance bottlenecks.
To make matters worse, GPU shortages have turned scaling generative AI applications into a game of musical chairs. Prices are skyrocketing, and wait times are longer than a DMV line. GPU capacity limitations have created additional hurdles for businesses looking to scale their generative AI applications. The scarcity of GPU resources has led to higher costs and longer wait times for access. This necessitates careful capacity planning, a practice not typically associated with traditional cloud services but now essential for ensuring consistent AI workload performance.
Capacity Options: Pick Your Poison
Organizations have two primary options when procuring capacity for AI workloads:
- Shared/On-Demand Capacity: It’s the “live fast, die young” approach—flexible, but with a side of unreliable performance when everyone’s trying to grab a slice of the pie. This pay-as-you-go model offers flexibility but comes with the risk of unreliable performance during peak demand periods.
- Dedicated/Provisioned Capacity: The “slow and steady wins the race” option—consistent performance and guaranteed resources, but you’d better be ready to commit long-term and fork over some serious cash upfront. This option guarantees consistent performance with reserved access to resources but requires long-term commitments and higher upfront costs.
Choosing between these options involves complex tradeoffs between cost and performance. In essence, it is somewhat like navigating a minefield—one wrong move, and your costs can blow up in your face. Dedicated capacity might keep things running smoothly, but you could end up paying for resources you don’t need when demand takes a nosedive.
Gunfight at the Token Corral
Generative AI services introduce an additional layer of complexity through token-based billing. Tokens represent the data processed by an AI model, with different models consuming tokens at varying rates. This abstraction complicates cost management, as predicting token consumption over time becomes challenging, making it difficult to align budget forecasts with actual usage.
Best Practices for Managing Cloud Costs in the Age of AI
To effectively navigate these challenges, organizations should adopt several best practices:
- Regular Load Testing: Constantly put your AI workloads through their paces to make sure they can handle whatever’s thrown their way without blowing your budget.
- FinOps and Engineering Collaboration: Get your financial gurus and machine learning masterminds working hand-in-hand to optimize resources and keep costs in check.
- Failover Logic Between Capacity Types: Make sure you’ve got a failsafe to switch between dedicated and shared capacity when the going gets tough.
- Incremental Scaling: Starting with small-scale experiments before committing significant resources helps identify potential issues early and reduces financial risk. Dip your toes in the water with small-scale experiments before diving in headfirst—it’ll save you from a world of financial hurt.
Blazing Trails and Managing the Books: Balancing AI Innovation and Cost Management
In the era of generative AI, organizations must adopt a proactive approach that balances the pursuit of innovation with robust financial oversight. Key strategies include:
- AI-Specific Cost Management: Implementing AI-centric cost management practices, such as real-time usage tracking, performance metrics analysis, and cost allocation tagging, is essential for optimizing spend.
- Collaborative Governance: Establishing clear governance frameworks that foster collaboration between FinOps, engineering, and business teams is critical for aligning AI initiatives with organizational goals and ensuring responsible AI adoption.
- Continuous Optimization: Regularly reviewing and adjusting cost management strategies based on evolving AI landscape and business needs is crucial for maintaining a competitive edge.
- (Optional) A Note on the Multi-Cloud, Hybrid Cloud Shuffle: As costs climb and flexibility becomes the name of the game, more and more organizations are embracing the multi-cloud or hybrid cloud tango. By spreading workloads across multiple cloud providers or mixing and matching public and private infrastructure, businesses can strike a balance between performance and cost while avoiding vendor lock-in.
Conclusion
The transformative power of generative AI is both a blessing and a curse, offering unparalleled opportunities while presenting new challenges in cost management and operational complexity. But fear not, intrepid adventurers! By embracing best practices like load testing, collaborative governance, and continuous optimization, you can harness the power of AI while keeping costs in check and staying ahead of the competition. The key is to adopt a holistic approach that brings together people, processes, and technology, empowering your business to thrive in the wild west of generative AI.
Related Blogs
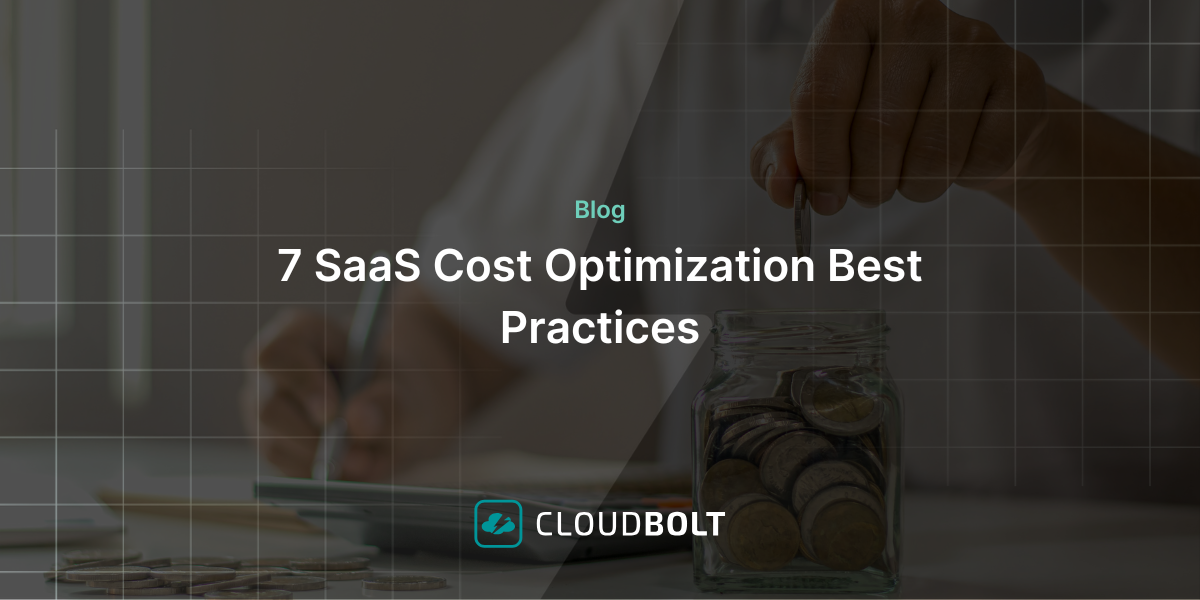
7 SaaS Cost Optimization Best Practices
The Software as a Service (SaaS) industry continues its robust expansion, significantly reshaping business operations on a global scale. In…